Blog
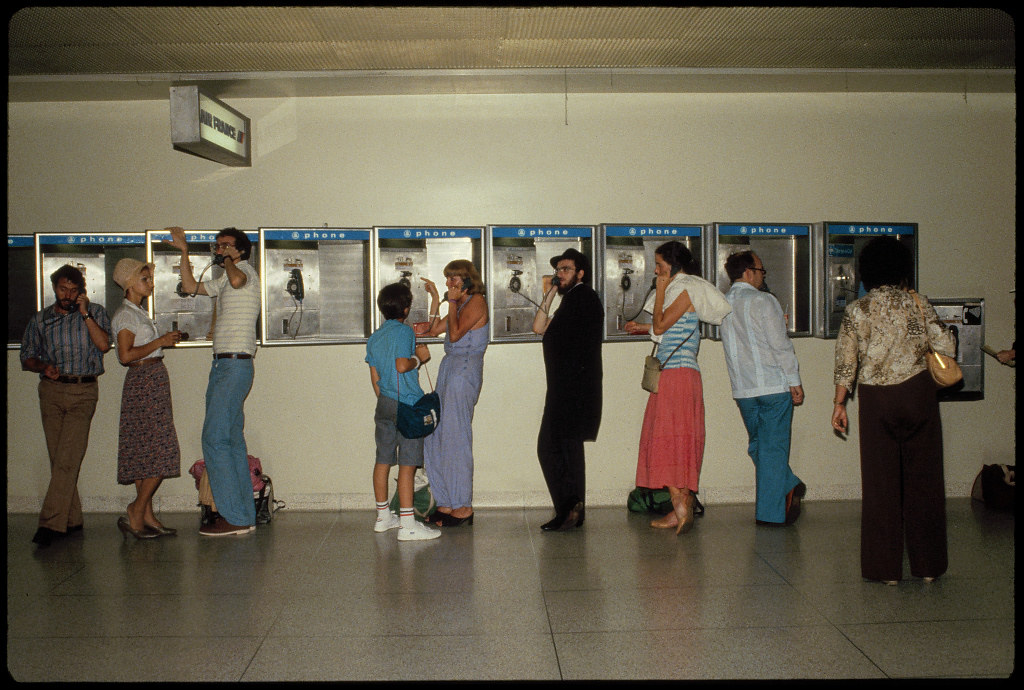
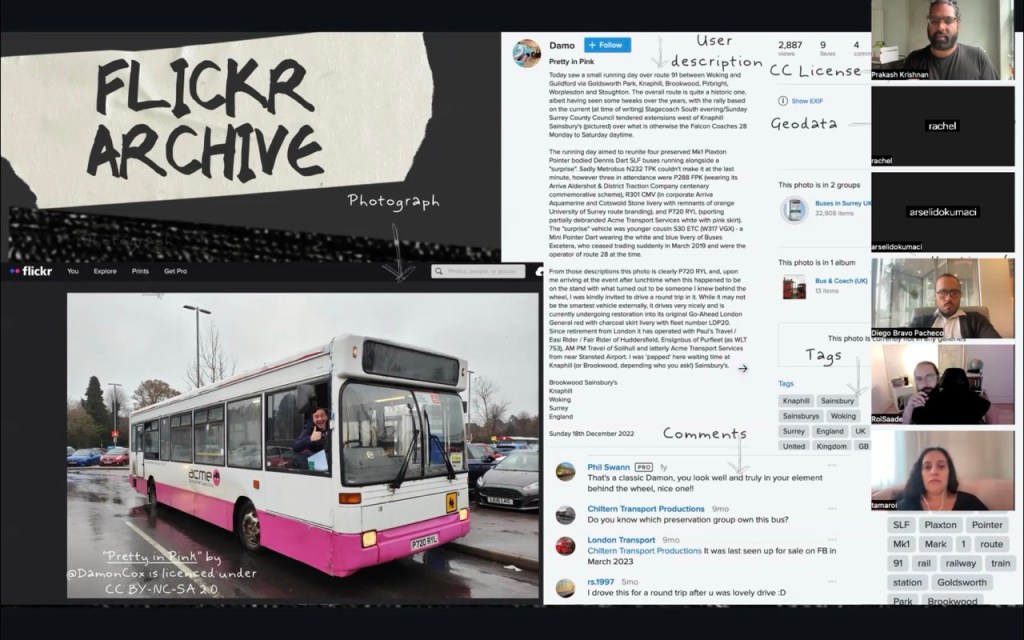
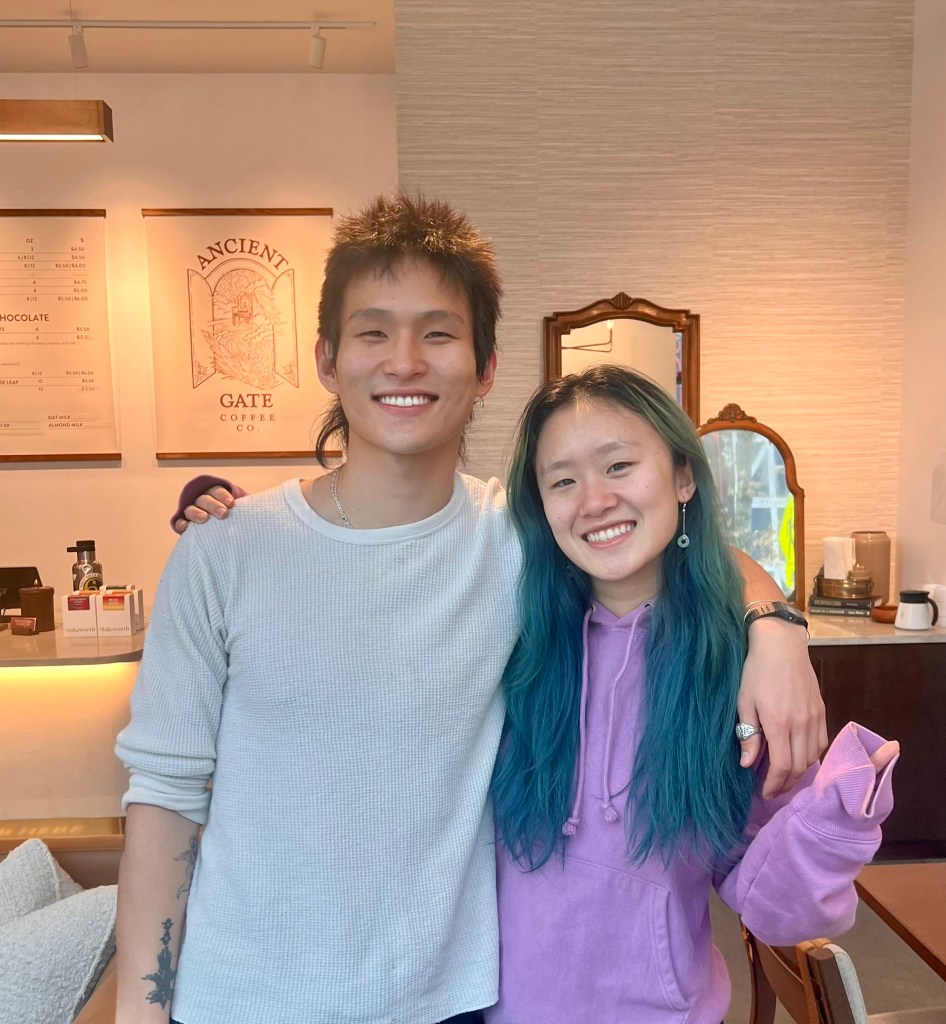
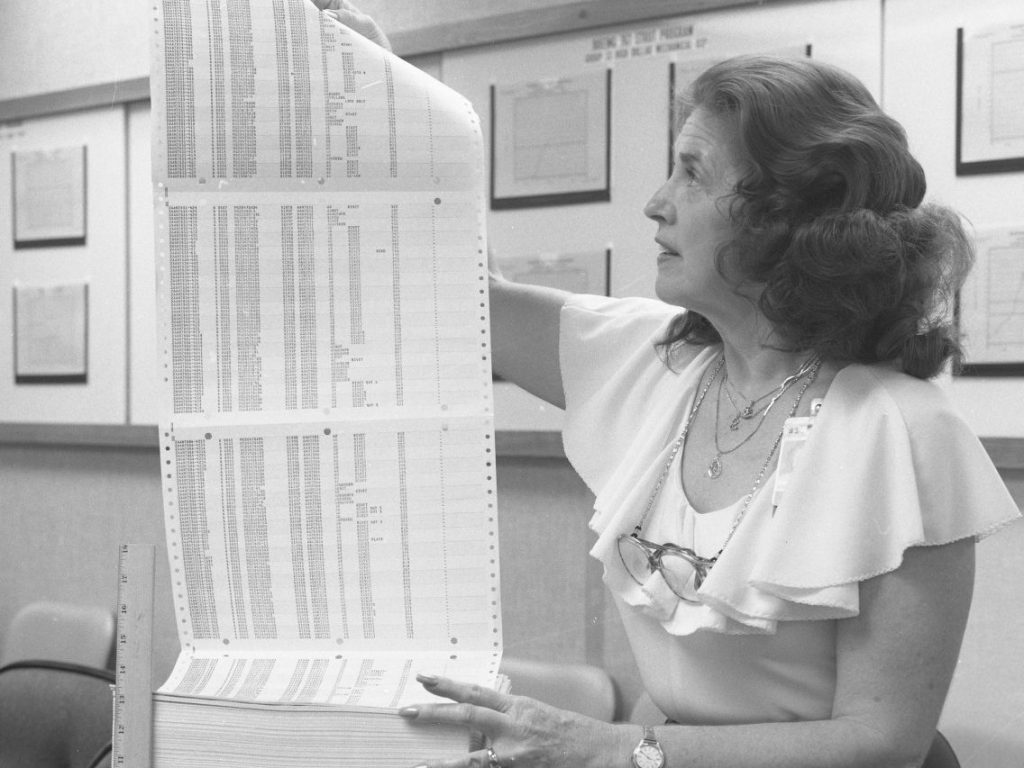
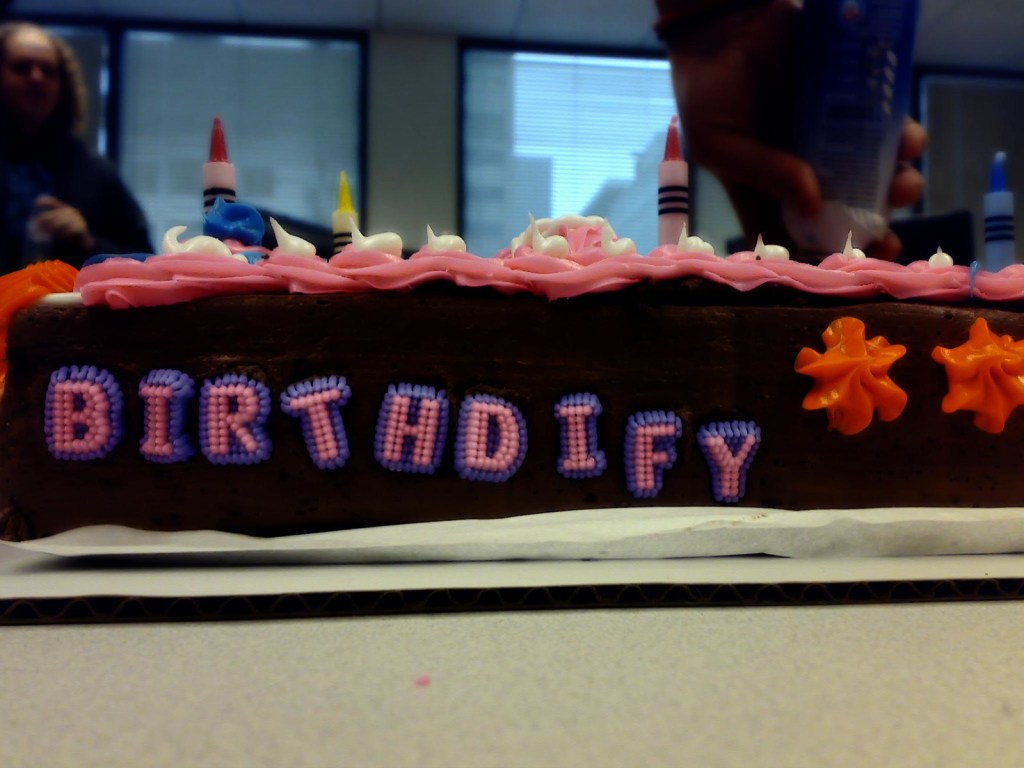
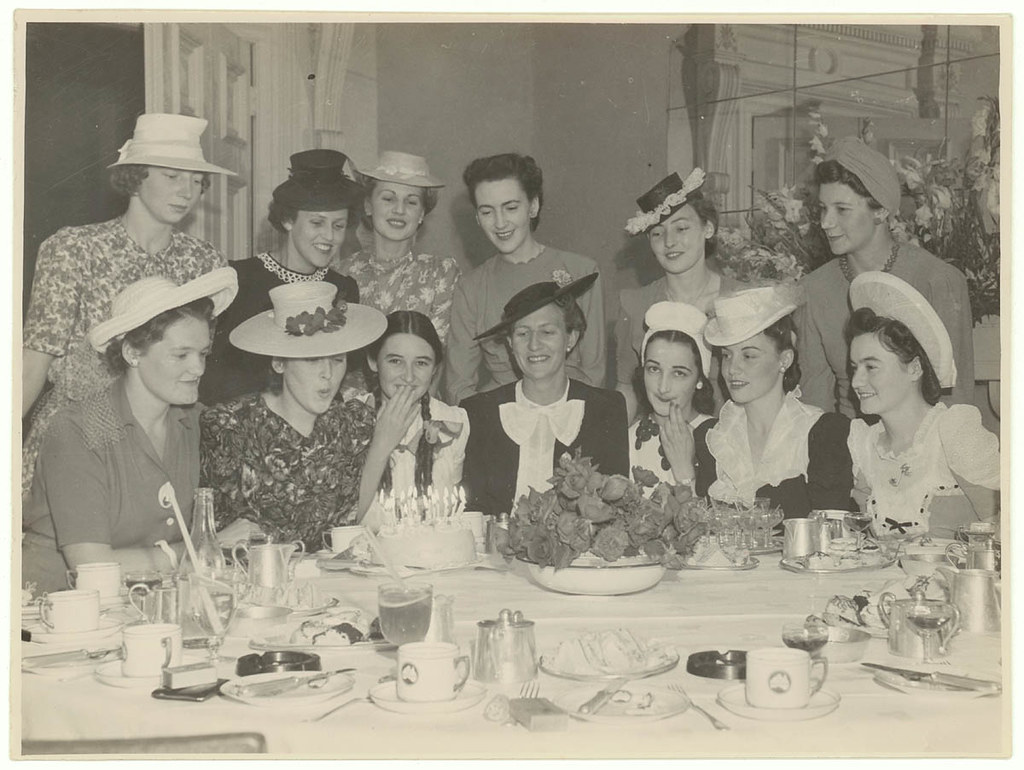
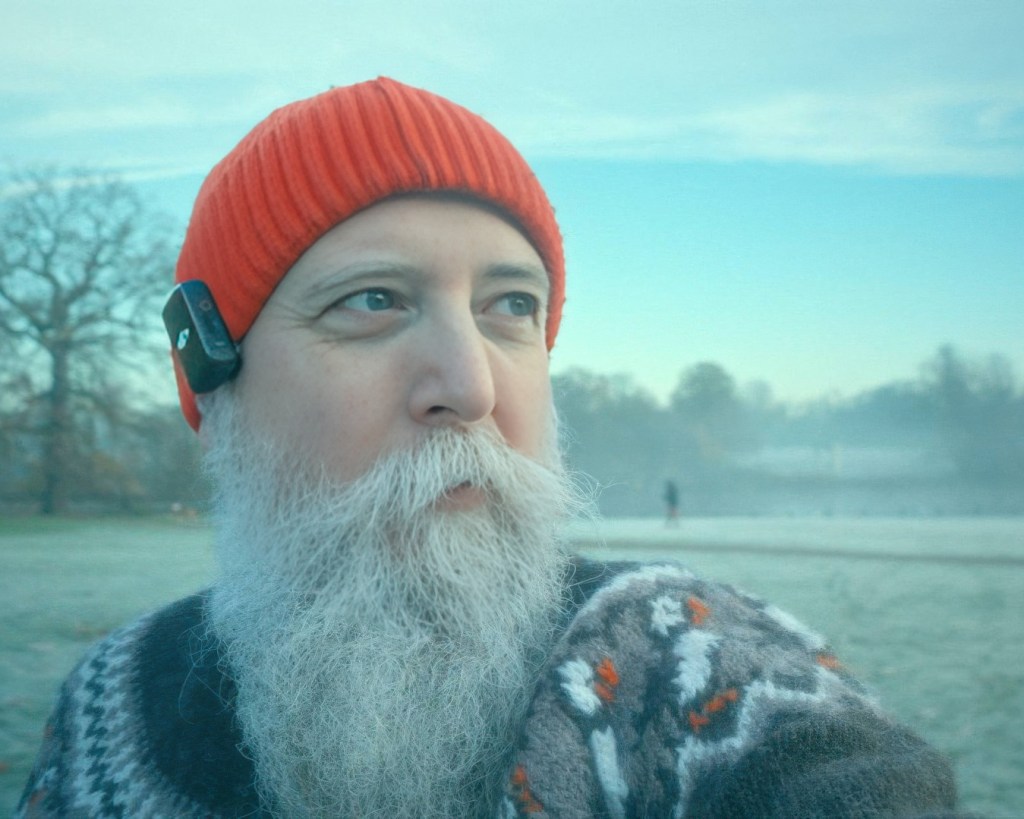
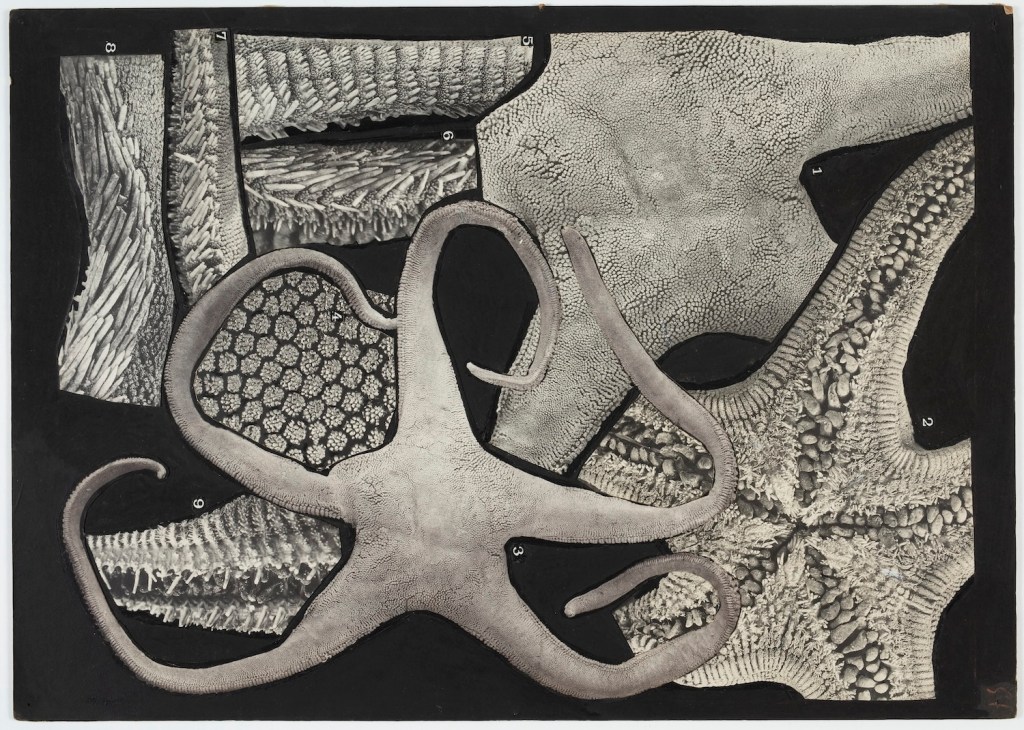
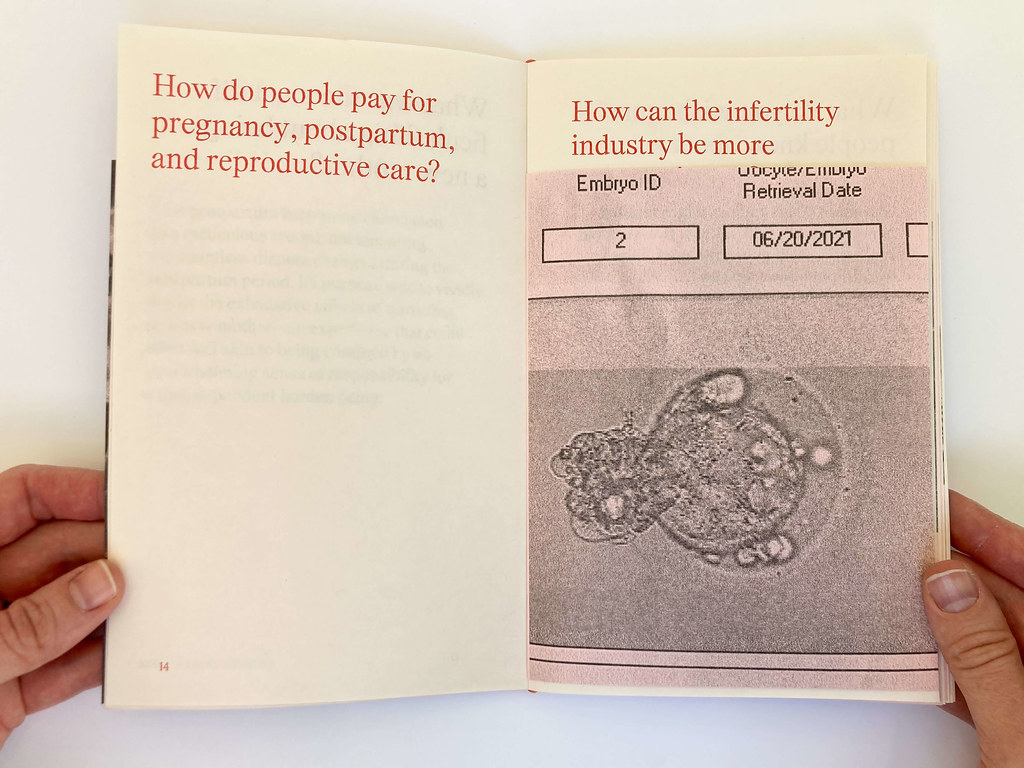
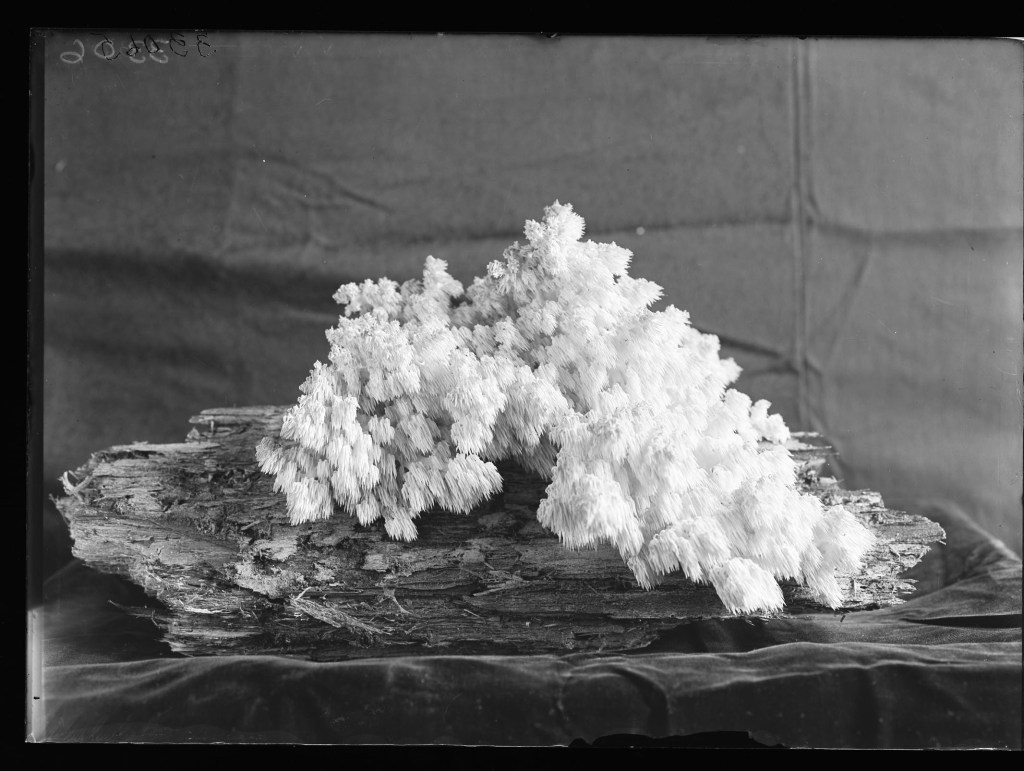
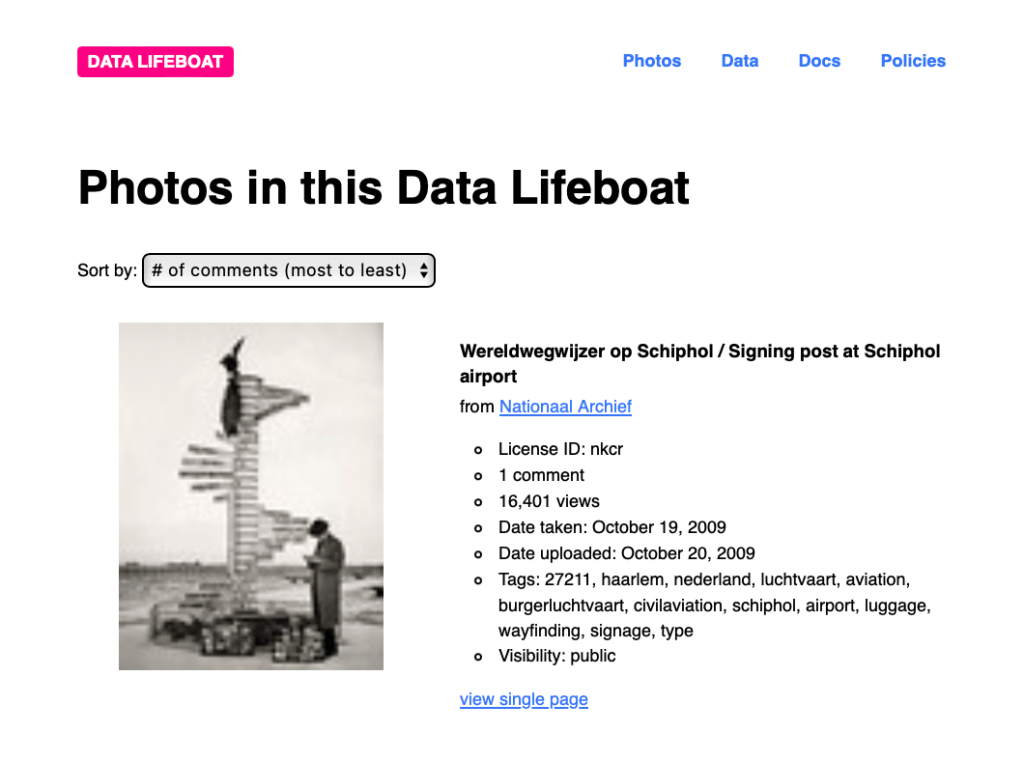
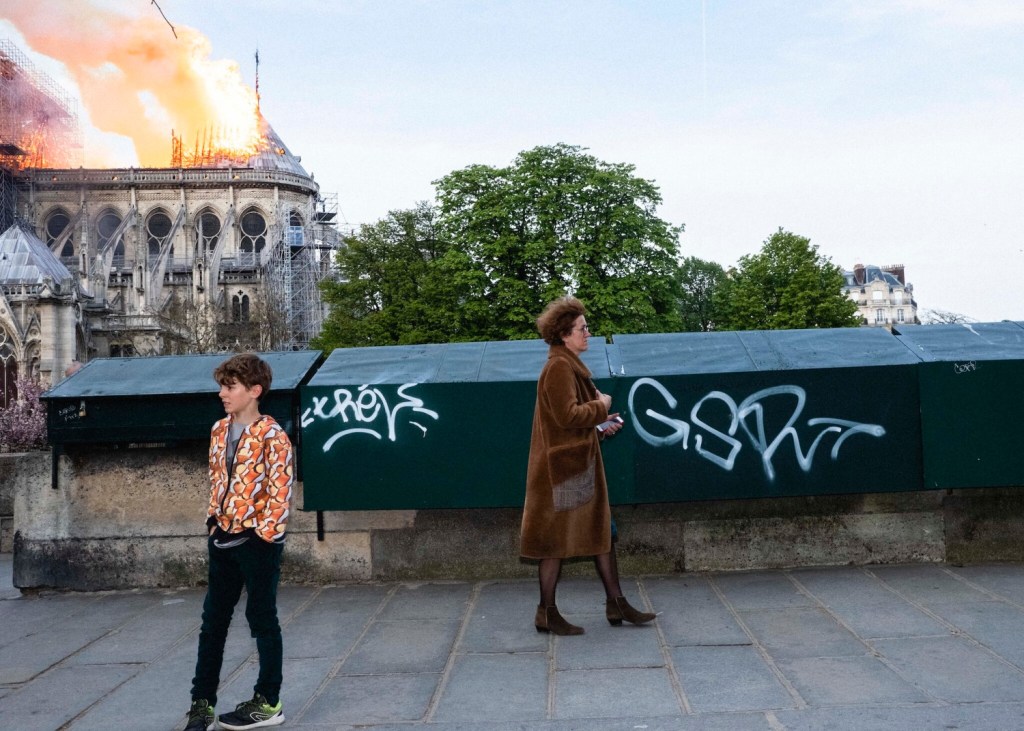
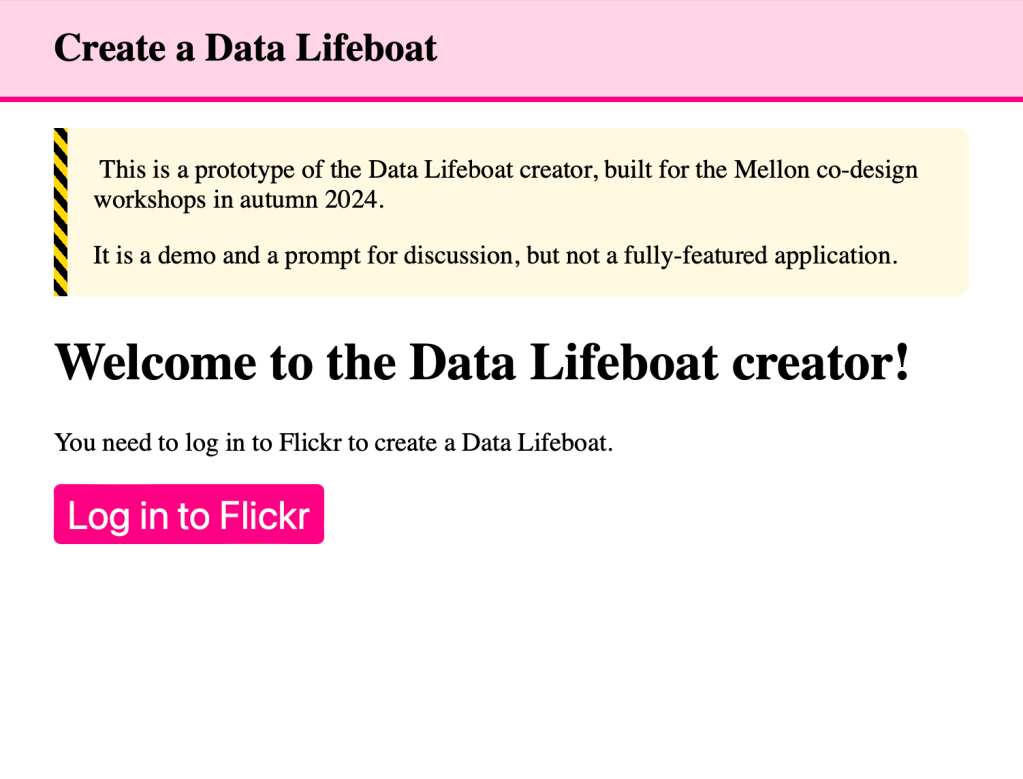
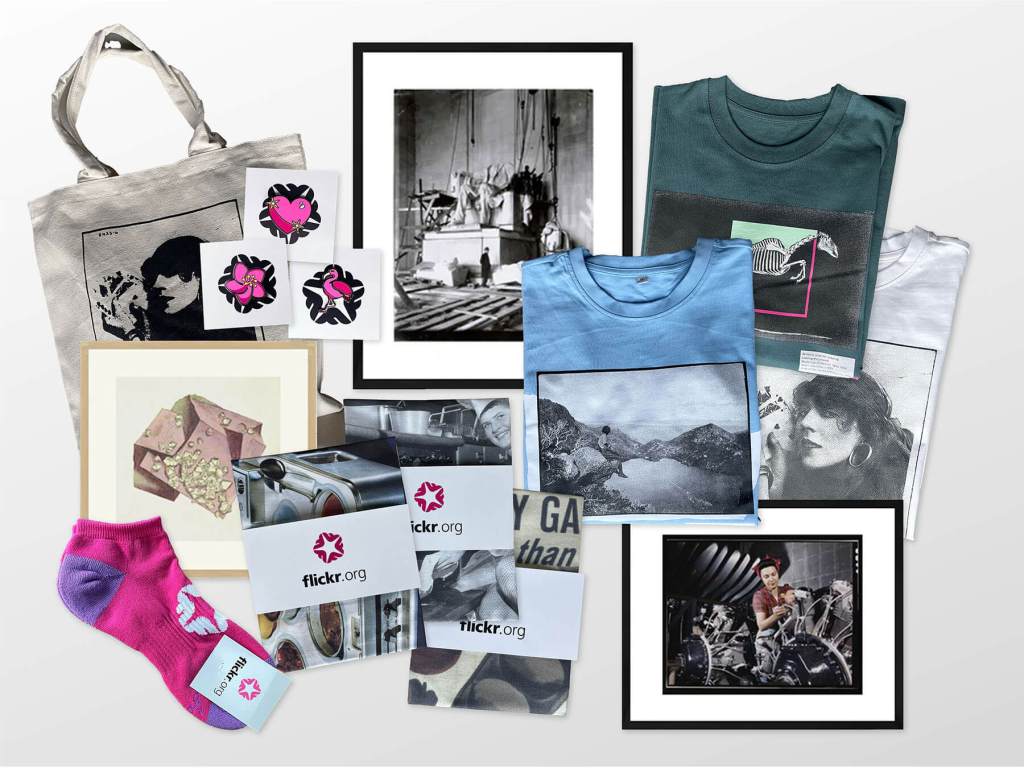
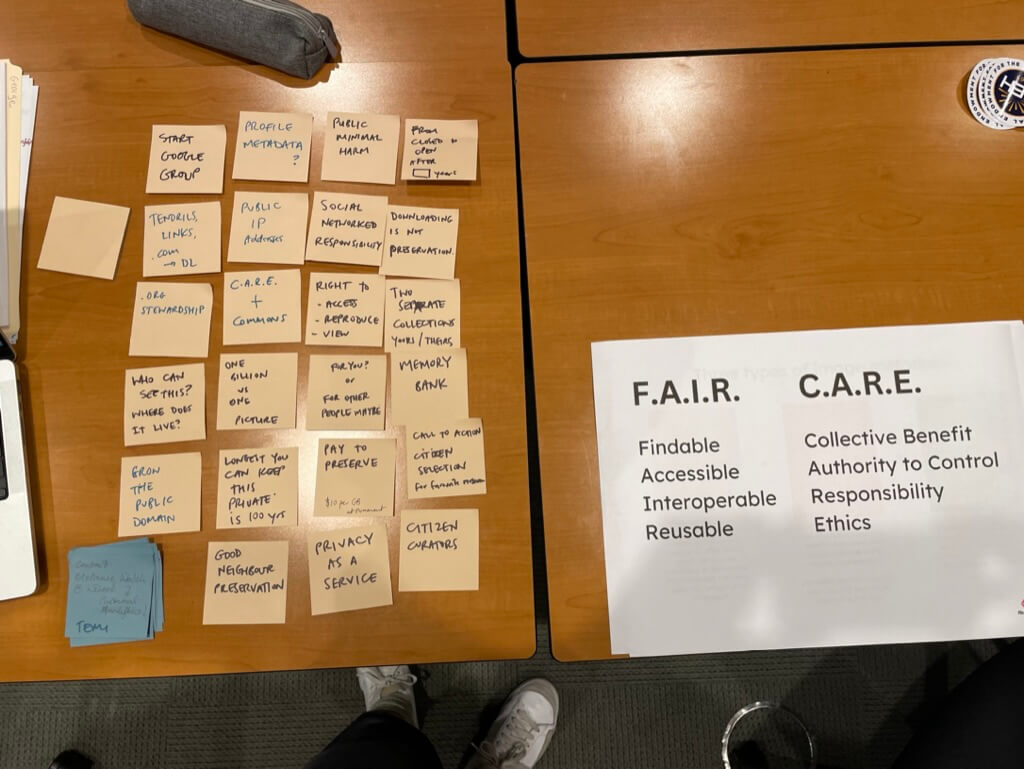
In the second instalment, Amy and Daniel discuss the expert interviews and user research they undertook to uncover insights into Gen Z's digital archival literacy
Meet the researchers Prakash ran his first Archivevoice workshop with, and their fantastic projects.
University of Washington Informatics students, Amy and Daniel, introduce their capstone research into digital legacy practices among young adults
An introduction to the rituals of record-keeping that have influenced our daybook project
Our flagship program, Flickr Commons, turns 17 years old today!
Dan has joined the team as Tech Co-Lead to work alongside Alex. We couldn't be happier!
The second of a two-part blog post detailing possible prompts for Data Lifeboat creators to encourage ethical and informed collecting
Emily Fitzgerald & Molly Sherman are joining us as our first research fellow pair to continue development of their Reproductive Reproductions project.
The first of a two-part blog post detailing the origins and approaches to ethical archiving in the Data Lifeboat tool.
See what you get when you download a prototype Data Lifeboat.
The case for citizen-driven collections in enhancing our understanding of contemporary events.
Check out our prototype workflow for creating a Data Lifeboat.
Take a look at our new online store, and get yourself a treat to support the Flickr Foundation
Now we are working out what just happened, and here's an outline.
Cookie | Duration | Description |
---|---|---|
cookielawinfo-checkbox-analytics | 11 months | This cookie is set by GDPR Cookie Consent plugin. The cookie is used to store the user consent for the cookies in the category "Analytics". |
cookielawinfo-checkbox-functional | 11 months | The cookie is set by GDPR cookie consent to record the user consent for the cookies in the category "Functional". |
cookielawinfo-checkbox-necessary | 11 months | This cookie is set by GDPR Cookie Consent plugin. The cookies is used to store the user consent for the cookies in the category "Necessary". |
cookielawinfo-checkbox-others | 11 months | This cookie is set by GDPR Cookie Consent plugin. The cookie is used to store the user consent for the cookies in the category "Other. |
cookielawinfo-checkbox-performance | 11 months | This cookie is set by GDPR Cookie Consent plugin. The cookie is used to store the user consent for the cookies in the category "Performance". |
viewed_cookie_policy | 11 months | The cookie is set by the GDPR Cookie Consent plugin and is used to store whether or not user has consented to the use of cookies. It does not store any personal data. |